Many of the RFPs we receive from our clients include requests for “conjoint analysis.” Unfortunately, this term and methodology can be ill-defined or not appropriate to meet the objectives of the research request. As researchers, it is our responsibility to help our clients better understand what they need and the best tools to employ to meet those needs.
>>Download our latest conjoint analysis eBook here.
Conjoint analysis offers a powerful set of tools that can quantify a number of metrics, including product or feature value; trade-offs customers are willing to make; and, in some cases, price elasticity for a product, feature, or brand. Clients and researchers have three core conjoint products to choose from:
- Adaptive Conjoint Analysis (ACA)
- The original replacement for traditional, card-sort conjoint
- Choice-Based Conjoint (CBC)
- The most popular, widely-used conjoint methodology
- Adaptive Choice-Based Conjoint (ACBC)
- Combines elements of both ACA and CBC
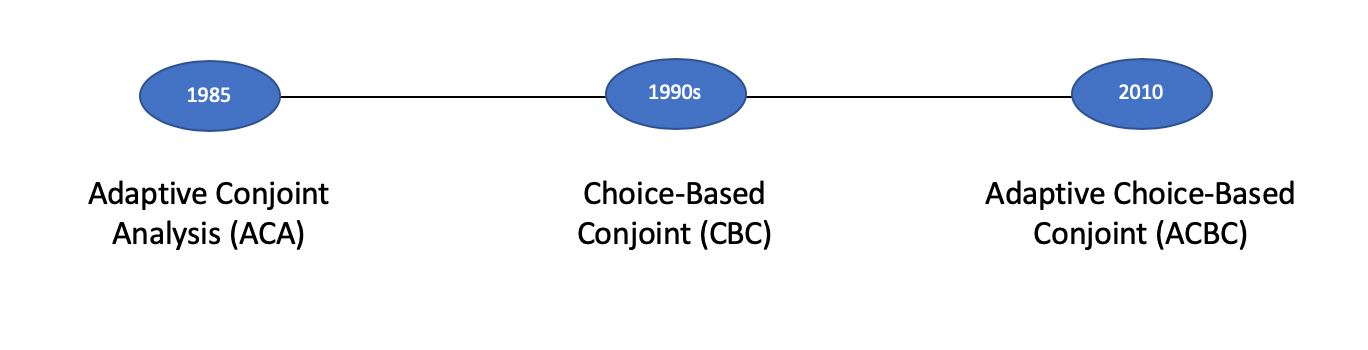
Adaptive Conjoint Analysis was released by Sawtooth in 1985. The ACA model was designed as a computer-based tool to replace traditional card-sort conjoint. It enabled researchers to measure more attributes than they could with traditional conjoint methods, making ACA a popular option due to ease of use and more powerful analysis.
Next, Choice-Based Conjoint was released in the 1990s and quickly became the most popular choice. This method eliminated attribute ratings and rankings, instead showing full “product” profiles from which respondents must choose. When companies want to better understand how people make choices when facing challenging trade-offs, CBC is the most widely-used survey-based approach. CBC also is known as discrete choice modeling (DCM) or discrete choice experiments (DCE).
Adaptive Choice-Based Conjoint is a newer methodology that was introduced around 2010. ACBC combines elements of CBC (Choice-Based Conjoint), artificial intelligence, and (optionally) dynamic list-building. It tends to probe more deeply into each respondent’s decision structure than traditional CBC. ACBC “combines the best aspects of adaptive interviewing with the realism and accuracy of choice data” (Orme, Brian. Getting Started with Conjoint Analysis).
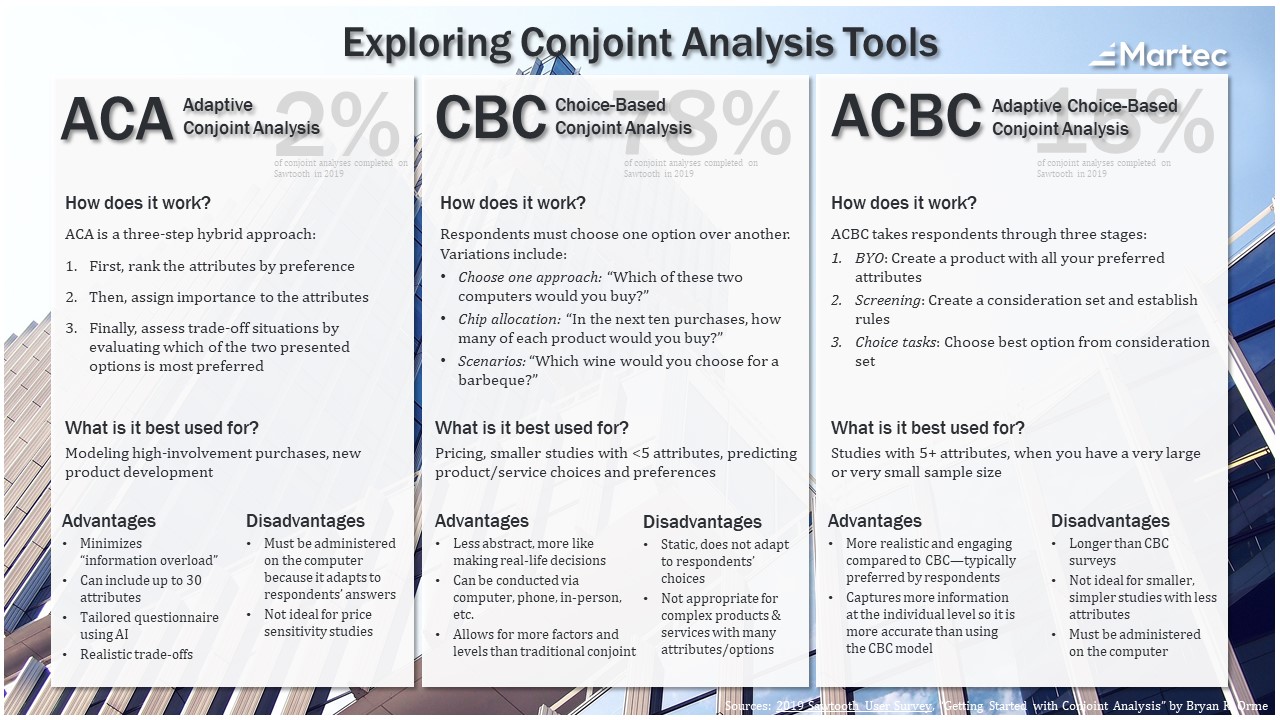
Still confused as to which research method is best for you? Contact us for a free consultation.
Related reading: A Brief Primer for Adaptive Choice-Based Conjoint